With the rising cost of living affecting almost every person and business on the globe, it’s no surprise that retailers are taking a massive hit. Can analysing key data and trends in changed customer behaviour help retailers withstand the effects of the cost of living crisis and help them thrive both online and offline?
How is the cost of living affecting retail?
Inflation has hit a 41-year high, reaching 10.4% in February 2023. According to the Office of National Statistics (ONS), retail sales volumes fell by 0.9% in the three months preceding January 2023 when compared with the previous three months. Compared to the same period one year earlier, sales volumes fell by a hugely significant 5.7%.
McKinsey found that 81% of consumers have changed their behaviour because of current economic pressures. And this is being felt massively across the retail industry, from big clothing brands to food giants. Products considered as luxuries have now slid further down consumers’ shopping lists.
The ONS reports that consumers are also making more shopping trips but buying smaller amounts, indicating a “little but often” technique to limit food waste. Retailers are seeing more and more product returns. Loyalty programmes are growing in popularity, allowing consumers to reap the benefits of deals and reward schemes, while streaming subscriptions aren’t being renewed as consumers calibrate their new budgets.
Understanding how customers are now behaving is the key to adjusting the way we communicate with them.
How can understanding data and customer behaviour improve sales and footfall?
Here are some ways you, as a retailer, can use data to better understand your customers:
Customer segmentation
Customer segmentation is the process of separating customer profiles based on different factors, for instance, unique needs and expectations. You can segment customers across the following factors:
- Geographic - based on consumers’ physical location.
- Behavioural - based on products or content consumers purchase or show an interest in and how they interact with a website, app or business.
- Psychographic - based on consumer lifestyle choices and preferences.
- Technographic - based on what devices and software consumers use.
Segmenting customer profiles (e.g. for email marketing purposes) allows you to target customers based on location, past behaviour (e.g. how long it’s been since their last purchase), their likes and dislikes (i.e hobbies) and what technologies they use.
Tailoring marketing communications in this way raises the likelihood of consumers engaging with the content you’re sending to them, which in turn, increases ROI. And analysing customer data would enable you to accurately segment your customer profiles. Using common programming languages like Python allows you to analyse large amounts of customer data and segment customer profiles with ease. The result is a more targeted outbound marketing strategy for your business developed by data-driven decision making.
Recommender systems
A recommender system is an artificial intelligence algorithm which uses data to suggest certain products, content or services for customers, based on demographics like location, purchasing history, and behaviour. The importance of recommender systems in retail has grown extensively with the rise of online retail tycoon Amazon, maximising retailers’ understanding of their customer base and turning their user experiences into streamlined, personalised customer journeys.
By analysing online purchasing and engagement behaviour, you’re then able to use recommender systems to promote content or products your users have already expressed an interest in, helping you drive more sales.
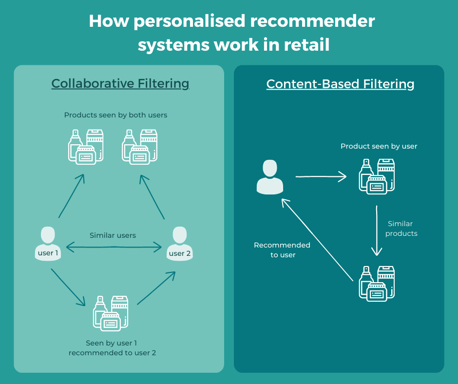
Retaining customer relationships
Data analysis can also help you identify at-risk customers to influence retention and repeat purchasing. According to McKinsey, 76% of people are more likely to consider a brand after receiving personalised communications. Customers want to feel heard and valued by the brands they shop at, without feeling like they’re just another transaction.
Analysing purchasing behaviour to find patterns across customers helps you build a more accurate model, or profile, of at-risk customers. You’re then able to send more targeted communications to entice those customers back into the purchasing cycle.
Personalised shopping experiences
Personalised shopping experiences don’t only apply to online customer journeys. Many high-end retailers like John Lewis and Harvey Nichols offer in-store personal shopping experiences.
Using customer data, such as previous purchases, could massively improve a customer’s in-store experience. Showing them products that they’re likely to have an interest in buying can make them feel important, listened to and can save them time, which today, is priceless to us all. Customers are much more likely to return to retailers where they have a seamless, professional and, ultimately, enjoyable experience.
Is upskilling in data analysis the answer?
Retailers are facing harsh challenges that have surfaced since the start of the Covid-19 pandemic. And like many retailers, you may want to implement some of the above solutions for improving sales but lack the necessary talent within your teams.
So how can you acquire the data skills needed to better understand these changes in customer behaviours? And equally important, how can your business afford to fund this talent acquisition in such a demanding hiring market? Government Levy-funded apprenticeships in data analytics could be the answer.